Machine learning exhibits remarkable learning abilities that can be harnessed to enhance blockchain systems. The trending blockchain technology offers a secure, decentralized system for direct peer-to-peer transactions, eliminating the need for intermediaries. Despite their inherent strengths, blockchain systems face certain limitations that machine learning can effectively address.
By integrating machine learning with blockchain technology, a synergistic effect can be achieved, resulting in high performance and practical outcomes. This article aims to delve into the fundamentals of blockchain technology and examine the integration of machine learning capabilities into blockchain-based systems.
Understanding Blockchain Technology
Blockchain serves as a securely shared ledger of decentralized data, enabling a specific group of participants to collectively share information. Through blockchain cloud services, transactional data from diverse origins can be effortlessly gathered, merged, and exchanged.
Data is segmented into interconnected blocks, each linked by distinctive cryptographic hashes.
This technology ensures data integrity by establishing a singular, authoritative source of truth, eliminating redundancies, and bolstering security. Within a blockchain framework, fraud, and data manipulation are prevented, as alterations require consent from a consensus of involved parties. The ledger remains shareable but immutable, triggering alerts for all participants if any unauthorized attempt is made to modify the data, revealing the perpetrator’s identity.
Read more: What Are Blockchain Layers
Exploring Machine Learning
Machine Learning, a subset of artificial intelligence, constructs algorithms by uncovering patterns within datasets. These algorithms are then employed to predict outcomes for new, comparable data without requiring explicit programming for each task.
Conventional machine learning integrates data with statistical tools to forecast outputs, providing actionable insights. This versatile technology finds application in various domains, including image and speech recognition, natural language processing, recommendation systems, fraud detection, portfolio optimization, and automated tasks. Moreover, machine learning models play a pivotal role in enhancing the intelligence and adaptability of autonomous vehicles, drones, and robots, enabling them to navigate changing environments effectively.
There are three major types of ML algorithms.
Supervised Learning Algorithm
Supervised learning is a category of machine learning where external guidance is necessary for the machine to learn. Models in supervised learning are trained using labelled datasets. After the training and processing phases, the model undergoes testing with sample data to assess its ability to accurately predict outcomes. The primary objective of supervised learning is to establish a mapping between input and output data.
Unsupervised Learning Algorithm
Unsupervised learning is a machine learning category where the machine does not require external guidance for learning from data. Unsupervised models are trained using unclassified and uncategorized datasets, and the algorithm operates on this data without any supervision. Unlike supervised learning, unsupervised learning does not have a predefined output for the model. Instead, it aims to extract valuable insights from extensive amounts of data.
Reinforcement Learning
Reinforcement learning involves an agent engaging with its environment through action and learning from feedback. The feedback manifests as rewards, with positive rewards for favourable actions and negative rewards for unfavourable ones. This learning process occurs without any external supervision provided to the agent.
Read more: Blockchain vs Crypto
The Intersection: Machine Learning and Blockchain
Machine learning (ML) can address scalability issues in blockchain networks. ML, a subset of Artificial Intelligence (AI), encompasses computer algorithms that autonomously enhance their performance based on experience and data. Regarding the scalability of blockchain, ML algorithms can be trained to anticipate transaction patterns, optimize network resources, and devise more streamlined consensus mechanisms. For example, ML can forecast periods of heightened transaction volumes, allowing proactive adjustments to network resources to accommodate increased demand, ultimately improving blockchain scalability. Furthermore, ML algorithms can contribute to the creation of more efficient consensus mechanisms, diminishing computational and energy requirements, and enhancing overall blockchain scalability.
The integration of machine learning techniques with blockchain technology not only enhances the efficiency and precision of blockchain systems but also elevates their performance. This fusion has the potential to refine the detection of fraudulent transactions within financial institutions. Moreover, machine learning can play a pivotal role in addressing security concerns in blockchain technology through diverse approaches such as supervised learning, unsupervised learning, and reinforcement learning. Additionally, the synergy of machine learning systems with blockchain technologies can safeguard the integrity of search results and protect sensitive data.
Potential Challenges and Considerations
There are certain challenges to overcome to improve their adoption; some vital ones are listed below.
Privacy
Information generated by devices and stored on the blockchain is open to all blockchain nodes, posing a potential privacy concern for data intended to be kept confidential. Employing private blockchains, implementing regulated access, and utilizing encryption can mitigate these issues. However, applying machine learning models to such restricted data introduces constraints on prediction and analytics capabilities.
Model Training and Network Latency
The primary challenge encountered in the application of machine learning algorithms is the insufficient quality and quantity of data. While data plays a crucial role in the functioning of machine learning algorithms, many data scientists claim that insufficient, noisy, and unclean data significantly strains these algorithms.
Regulatory and Ethical Implications
Blockchain users have raised concerns about insufficient regulations at specific stages of the process, leading to challenges. Nevertheless, the absence of clear regulatory requirements poses significant risks for both blockchain providers and consumers.
When developing AI systems, it is crucial to contemplate the ethical implications of the technology. Various methods can be employed to integrate ethics into AI systems, such as:
- Infusing ethical values into the design of AI systems
- Assessing the impact of AI systems on diverse groups of individuals
- Implementing regulations to govern the utilization of AI systems
Future Outlook and Trends
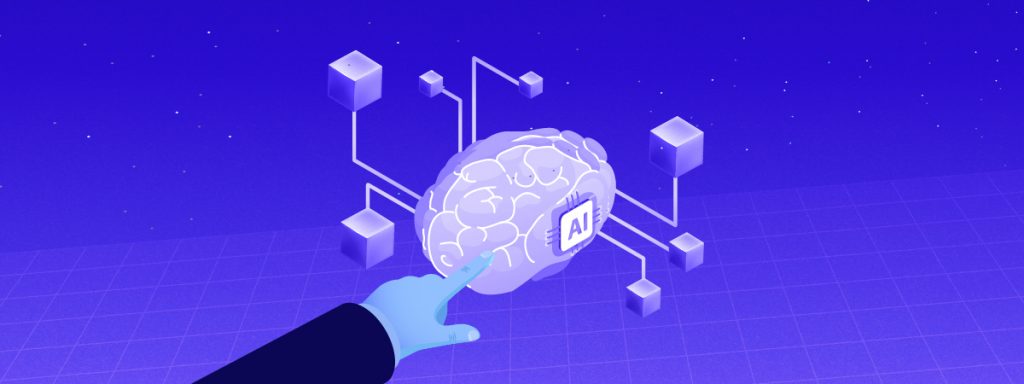
Machine learning presents diverse solutions to contemporary business challenges, encompassing methodologies such as deep learning and reinforcement learning, all integral to big data analytics. Concurrently, blockchain networks serve as a framework for storing and sharing significant volumes of data through smart contracts, creating an intersection of machine learning (ML) and blockchain-centred data credibility and data-driven decision-making.
In this symbiotic partnership, blockchain ensures data security, potentially fostering data sharing in ML model training and testing phases. Additionally, blockchain’s utility extends to allocating computing resources, establishing Internet of Things (IoT) networks, and constructing online predictive models using varied data sources.
However, the decentralized nature of blockchain systems, generating extensive data from diverse origins, poses challenges in tracking and controlling compared to centralized systems. Machine learning emerges as a solution to this issue, leveraging its predictive capabilities for system behaviours, efficient data analysis methods, and the enhancement of data verification processes. ML models prove instrumental in identifying malicious attacks and detecting fraudulent transactions within the blockchain.
Examining the interplay between these technologies reveals a transformative potential for reshaping our interaction with and reliance on data. The analytical prowess of machine learning, coupled with the decentralized nature of blockchain, represents a significant advancement in artificial intelligence. This fusion creates a trustworthy foundation for data-driven decisions, offering a more cohesive, transparent, and manageable approach to handling data.
Read more: Advantages And Disadvantages Of Different Types Of Blockchain
Conclusion
The intersection of ML and blockchain scalability is in its early stages but holds promise as a potential resolution to a significant challenge in the blockchain space. This prospect envisions an environment where the limitations of transactional speed and volume no longer impede the full potential of blockchain.
Is ML genuinely poised to be the solution for unlocking blockchain scalability? Encouraging signs suggest so, and as technological progress rapidly advances, it becomes more evident that the future of blockchain scalability is intricately tied to the capabilities of ML.
If you found this blog to be useful, do share it with other like-minded crypto enthusiasts. Click on the button below to begin your crypto trading journey using ZebPay.